CRACK CATCHER AI
Silicon (c-Si) solar cell technology remains the most cost-effective way to commercially harness solar power for conversion to electricity. However, silicon is a very fragile material, by nature. Crack will inevitably occur – it’s just a matter of time (and wind, and snow, or birds hitting them, or people stepping on them).
The current method of alleviating it is at best just trying to detect it by electroluminescence (EL). It’s too little too late. By the time cracks are detectable by EL method, they are “adult” cracks – they are already too big to halt. Cracks tend to keep propagating (ie. getting larger) as they exceed their critical crack size.
In Crack Catcher AI, we aim to stop cracks in silicon solar cells when they are still babies. Nip it in the bud. Before they achieve their critical crack size. We use fundamental Fracture Mechanics combined with the aids of Artificial Intelligence (AI). Enter CRACK CATCHER AI!
CCAI Summary
CRACK CATCHER AI – PROBLEM STATEMENT
Silicon (c-Si) solar cell technology remains the most efficient and powerful way to commercially harness the solar power for conversion to electricity. SunPower, a leading silicon solar PV company based in California, U.S.A., for example, leads the commercial market with the highest cell and module efficiencies, and the highest and most robust installed solar PV systems in the world. In order to keep improving the market adoption rate and thus providing solar energy that is cost-competitive with existing energy sources, the global silicon solar PV industry is aggressively reducing the cost of solar PV systems through innovations in materials and processes used in the manufacturing. One potential area of significant cost reduction is through using thinner silicon cells (towards 100 mm). However, coupled with other recent trends in the solar PV module technologies (thinner glass, new types of polymers for encapsulation of the cells), high stress and fracture of the silicon cells are increasingly observed and reported for products using thin silicon solar cell technologies, such as shown in Fig. 1 below.
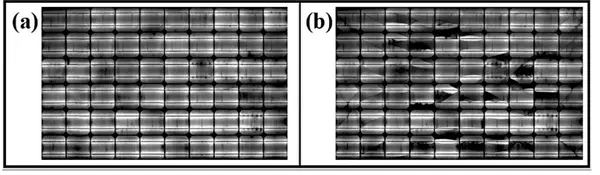
(to simulate wind load):
(a) Electro-luminescence (EL) image of the PV module before test and (b) after test*
Cell cracks immediately lower module efficiency and can lead to premature aging of the entire package/module. Silicon cell cracks have been observed since a long time ago, but only recently had it been observed more significantly during the cell and module high volume manufacturing – so not just later in the fields after installations. In other words, it is now no longer product reliability issue (or just warranty issue), but also manufacturing yields. This directly affects the bottom line and thus the economics of silicon PV high volume production, especially in the United States. Hence, our proposed project here directly contributes the cost-competitiveness of silicon PV module manufacturing capacities of our country.
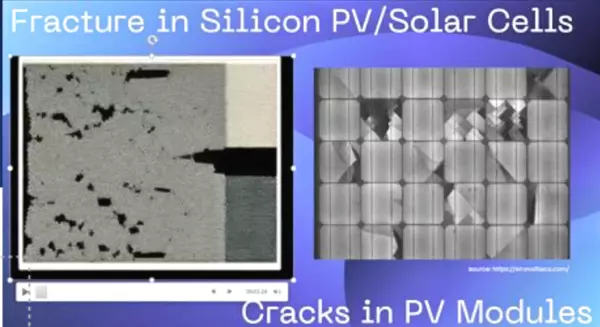
Figure 2. Fracture in silicon solar cells and PV modules.
Silicon is a brittle material and very fragile. In extreme weather like Oregon’s, cracks are
bound to happen in the fields due to wind, snow, hails, etc.
Many research groups in the world have been studying fracture mechanisms in silicon solar cells9-12,22-24 more systematically, especially since our own first report (collaboration between SunPower and Berkeley national lab) on the topic in 2014.9 However, those previous studies in the literature have been largely focused on the crack detection and imaging techniques.3-6,46-53 Lacking the capacity to quantitatively characterize mechanical stress, which is the ultimate driving force of any crack initiation and propagation, these studies did not reveal much fundamental understanding of what affects the propensity to crack, the various failure modes, and, most importantly, the processes and loading conditions that lead to different states of mechanical stresses that ultimately cause different modes of the cracks. Our work with synchrotron X-ray microdiffraction (µSXRD) has thus proved to be a suitable technique to elucidate stress and crack propensity in stringed silicon solar cell assemblies,9-12 as shown earlier in Figure 1(a). This technique provides quantitative determination of stresses in silicon and allows mapping of these stresses (normal, hydrostatic and shear) in the high stress concentration areas in the stringed cell assembly in the appropriate spatial resolution (up to a micron).9-11 The proposed stress sensing technique in the current proposal was inspired by this method but will not be using the synchrotron radiation. It will use high-resolution laser source instead but will be as quantitative as the synchrotron radiation technique, as was demonstrated comprehensively in Ref. 11. This approach can thus provide one with the quantitative examination of stress and its evolution during the processes and operations of the devices in the realistic setting.
Fracture in thin silicon solar cells, for instance, has been proposed to result from the high stress concentration area around the solder joints and tabs that provide interconnection between the cells (stringed cell assembly)9-12,45 such as shown earlier in Fig. 1(a). Studying how stresses especially in these areas evolve during introduction of the solder joints in the solar cell assembly lines has proven crucial in highlighting the propensity to fracture as well as its initiation and propagation mechanisms in the thin silicon solar cells. This proposed project aims to provide the high volume manufacturing the tools to solve this challenge in the silicon solar PV industry, and thus establish the United States’ supremacy in high technology manufacturing.
OUR SOLUTION
Fracture mechanics, especially in brittle materials such as silicon solar cells, is always, in the end of the day, highly probabilistic in nature.13-15 The fundamentals of fracture mechanics provide only two sides of the equation – first is the stress, which is the driving force of any crack initiation or propagation, and secondly the fracture strength of the silicon cells.13-15 With the silicon cells being thinner and thinner (towards 100 mm), 8 not only both sides of the equation are working against us in developing robust and reliable, next generation silicon PV systems, but also the statistical nature of both stresses and materials strengths at such thickness of the silicon cells16-17 are making fracture prediction (certainly in the design stage but also in high-volume manufacturing) increasingly more and more important, not only technologically (e.g. in enabling novel cell design or architectures) but also commercially (higher manufacturing yield means lower PV system cost to the customers).
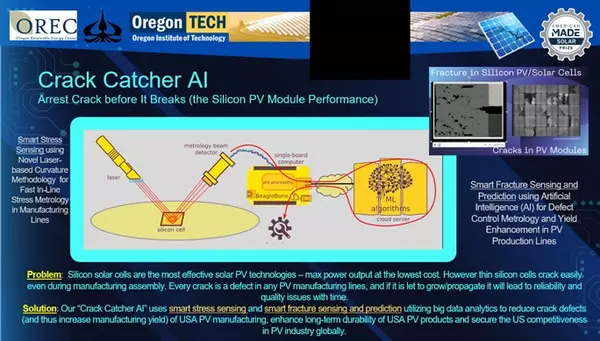
Fundamental Fracture Mechanics meets Artificial Intelligence (AI)!
Mechanical stresses are the driving forces of fracture events, thus the exact knowledge of stress – its highly localized concentrations and overall distribution in the solar cells (according to cell design/architecture, etc.), its evolution in the manufacturing sequences and its constant negotiation with the strengths the materials could provide at a given position in space (ie. across the cells) and in timeline (ie. along the sequences in the cell manufacturing as well as its integration into a PV system) – provides an important predictive ability of fracture occurrences. Built on our existing research expertise in enabling thin silicon solar cell technology, we aim to develop a smart stress sensing for inline production metrology tool, based on the local curvature technique18 (using in principles, still the same Stoney’s equation,19 but translated to much more local curvature11,20 information in continuous thin film) and enabled by high-resolution laser technology. This smart stress sensing system would provide the knowledge of stresses in each of the silicon solar cells in the manufacturing line and how they evolve during critical processes (ie. yield-loss making in terms of cell breakages). Such a smart stress sensing has not been hitherto possible in the present high-volume manufacturing environments (so far, only in synchrotron labs, such as reported by our group11 recently).
However, we do not aim to stop there. Given the highly probabilistic natures of both stresses and materials strengths,13-17 especially at such a highly fragile geometry, and thus the rather complicated negotiations between the two sides of the equation (with respect to positions in the solar cells, as well as to time during the manufacturing sequence), what better tools to predict such fracture occurrence in thin silicon solar cells than using AI (Artificial Intelligence) and data analytic approach? Thus, we aim to develop also smart fracture sensing and prediction – basically a smart Industry 4.0 Advanced Manufacturing system that could predict or sense if fracture is about to occur in the silicon solar cells in the high-volume manufacturing environments. The smart fracture sensing would enable the global PV industry to realize the thin silicon cell (towards 100 micron) advantage, which would in turn, lead to USA PV manufacturing competitiveness, through Advanced Manufacturing methodologies.
In this proposal, we will hence undertake fundamental and demonstrative research and development (R&D) to push boundaries in key technology areas related to Smart Stress Sensing and Smart Fracture Sensing to enable design and high-volume manufacturing of next generation, thin but robust/reliable silicon solar PV systems/technologies. To achieve these objectives, by the Set! Phase of the competition, we will have developed lab scale or pilot line-scale prototypes that provide the proof of concepts for the two interrelated systems:
- The Smart Stress Sensing Systems – based on the high-resolution laser instrumentation and enabled by the local curvature technique (Stoney’s equation). This instrumentation technique will be used to design and manufacture next generation, thin but robust and reliable silicon PV systems.
- And the Smart Fracture Sensing Systems – based on AI and big data analytics. This system will be used for an in-depth understanding and thus for a predictive ability of the fracture behavior of thin silicon PV systems and materials.
We leverage the resources that we get from the members of the American-Made Network, and solar PV industry and manufacturing partners. Our team is in close contact with many existing industry collaborators in SunPower 9-10,22-23 (San Jose, Bay Area), GAF Energy 9-10 (San Jose, Bay Area) and REC Group 24 (REC-USA).
Smart Fracture Sensing
This part will be the development of a system for data collection, data delivery to a cloud infrastructure and performance of statistical analysis of the initial data set based on the local curvature measurements system. The curvature inline metrology measurements system will be ready to integrate into high-volume c-Si PV manufacturing system by delivering data in real-time to an intermediate computing (a SoC – System on Chip – computer) system as illustrated in Fig. 4.
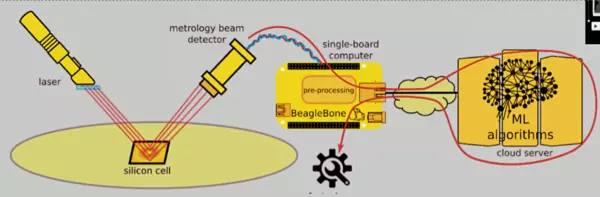
data processing via BeagleBone and big data analysis by cloud computing machine algorithms
To manage cloud computing and big data analysis challenges for the incoming detected values the processing should be done by statistical classification methods like: support vector machine,34 self-organizing feature maps,35 k-nearest neighbours,36-37 decision trees,38-39 neural networks,40 or other algorithms and their modifications.41-42 In order to process measurement results in this manner, it is necessary to have some samples, however it is difficult to estimate the order of the number of samples which are necessary for a first evaluation of the given problem. However, the lack of training data is a common problem in machine learning,43 it can be solved with data augmentation technique,44 over which we can intentionally distort available data to extend training sample set. After acquiring probability and setting reasonable limits that measured data corresponds to the inline detected samples, the estimation of the surface curvature becomes possible. The development of trained algorithm will allow to handle and use the data during the production process on-the-fly.
OUR TEAM
Our group (PI Budiman) has been studying the mechanical stress and thus fracture mechanisms in thin silicon solar cells9-12,22-24 more systematically since our first report in 2014.9 It has since thus increasingly become both an interesting topic scientifically as well as an important subject technologically during recent years especially with the oncoming development of the new generations of solar PV renewable energy systems. The methodology for smart stress sensing here thus will expand and build on PI Budiman’s existing framework of solar PV materials/industry research.
The smart fracture sensing will be built on the strengths of existing collaboration with our Indonesian partners – Dr. Endang Djuana and Dr. Henry Candra from Smart Network and Industrial Automation Lab, Trisakti Electrical Engineering Department. The Trisakti AI Team will bring along the combined expertise in machine learning. Dr. Djuana has implemented the Deep Learning with Greedy Layer-Wise Compound Scaling for Temperature and Humidity Prediction in Solar Dryer Dome. He has also researched designing Smart Dome 4.0 with a Low-Cost, Independent, Automated Energy System for Agricultural Purposes by implementing the Machine Learning algorithm. Dr. Candra has conducted research by implementing the machine learning algorithm in biomedical engineering to recognize the emotion from the recorded EEG signals and facial expressions using a support vector machine, hybrid fuzzy cognitive, and deep learning algorithm using a Convolutional Neural Network.
Dr. Budiman (Oregon Renewable Energy Center/OREC and Oregon Institute of Technology/OIT, and formerly of Singapore University & Technology/SUTD) and Dr. Endang Djuana (Trisakti University, Jakarta, Indonesia) have also been collaborating closely in the past few years – our shared research interests in the machine learning techniques to optimize Renewable Energy systems had brought us even closer recently in the forms of student exchanges and existing collaboration research grants (sponsored by both the United States and the Indonesian governments). Our team has the capability for designing and developing the low-cost, localized, self-sufficient energy systems based on solar photovoltaics (PV)54-56 and the proven expertise and experiences of implementing deep learning methodologies for such PV energy system.
OIT has the facilities of Renewable Energy Engineering (REE) Laboratories as well as the Academic Programs at the Klamath Falls Campus. The laboratories are facilities for OREC, in which PI Budiman is currently the Director. Dr. Derrick Speaks and Prof. Tim Pasang (along with PI Budiman) are all faculty members of OIT in Klamath Falls, OR. Prof. Pasang is the Head of Department of Manufacturing and Mechanical Engineering & Technology (MMET) both based in Klamath Falls. Our joint collaboration team has the proven expertise and experiences to realize the objectives and the vision of the current plan. The methodologies and approaches proposed in this document are appropriate, and many infrastructural set up (equipment, analytical tools, testing capabilities, etc.) needed for the proposed work are available within our campuses.
WE WON AMERICAN-MADE SOLAR INNOVATION CHALLENGE 2022
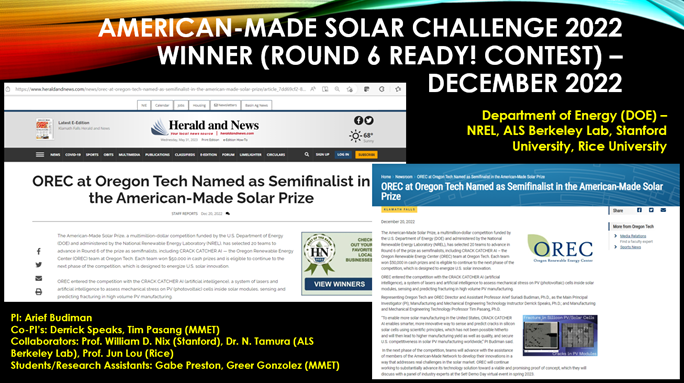
Important Vocabulary
Electroluminescence – a low power consumption technology that excites electrons where they release their energy as photons (light). The electroluminescence is the result of the radiative recombination of the electrons and semiconductors on the solar cell, improving the light to electricity ratios of the solar cells (aka, electron interactions with semiconductors withing an electroluminescent film produces maximum efficiency in solar cells).
Fundamental Fracture Mechanics – Mechanics of solids which contain planes of displacement (cracks) and how they grow using energy.
Mechanical Stress – A physical quantity describing internal forces of an object that resist the external forces applied to it.
Synchrotron X-ray Microdiffraction – Provides direct structural information leading to the identity, crystallinity, and potential impurities of a crystal structure. By providing the information, it enhances the fluorescence and absorption fine structure measurements.
Elucidate – to make something clear or explain
Propensity – a natural tendency of body to behave in a certain way
Stoney’s Equation – a formula that relates differences in surface stresses between a chemically modified surface and the untreated surface with a concave down bending force.
Inline Production Metrology – A process of automatic measurements taken and used during the manufacturing process. (i.e. the parts are note removed form the manufacturing line for manual inspection)
Data Analytics – Collection, transformation, and organization of data to form hypotheses and draw conclusions.
Support Vector Machine – Supervised learning models with related algorithms that analyze data for classifying and regression analysis. It is used on machine learning.
Self-Organizing Feature Maps – competitive neural networks
K-nearest Neighbors – A basic and essential classification algorithm used in machine learning used for pattern recognition, data mining, and intrusion detection.
Decision Trees – A hierarchical model showing decisions and their possible consequences. This includes chance event outcomes, resource costs, and utility.
Neural Networks – when neurons ae organized in a one dimensional lattice grid that represents the feature space. The grid characterizes positions of neurons and gives exact geometric locations.
Machine Learning – An umbrella term for solving problems from using machines to “discover” their own algorithms to solve problems.
Data Augmentation Technique – Methods commonly used in deep learning domains.